Paper presented in ISM 2019
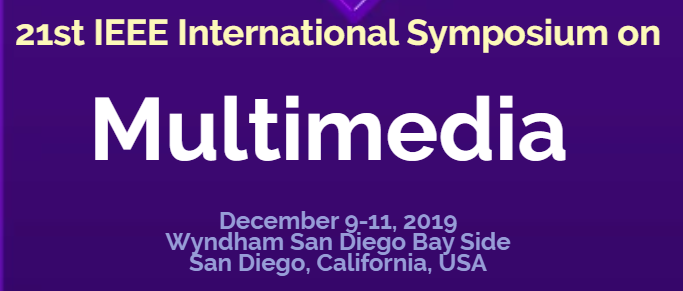
Machine learning based applications that run on image datasets increasingly use local image feature descriptors. We can visualize images as objects and local features as parts. Typically, there are thousands of local features per image, resulting in an explosion of feature set size for already huge image datasets. In this paper, we present a feature set consolidation strategy based on two aspects: pruning of non-discriminatory features across different object types and association of matching features for the same type of objects. We showcase the effectiveness of our consolidation strategy by performing classification on a building dataset. Our method not only reduces storage space footprint (~5%) and classification runtime (~4%) but also increases classification accuracy (~2%).
Piyush Yadav, Shamshuddin Ladha, Shailesh Deshpande, Edward Curry, “Feature Set Consolidation for Object Representation by Parts”, In 21st IEEE Symposium on Multimedia (IEEE ISM ’19), San Diego, California, USA, 2019.